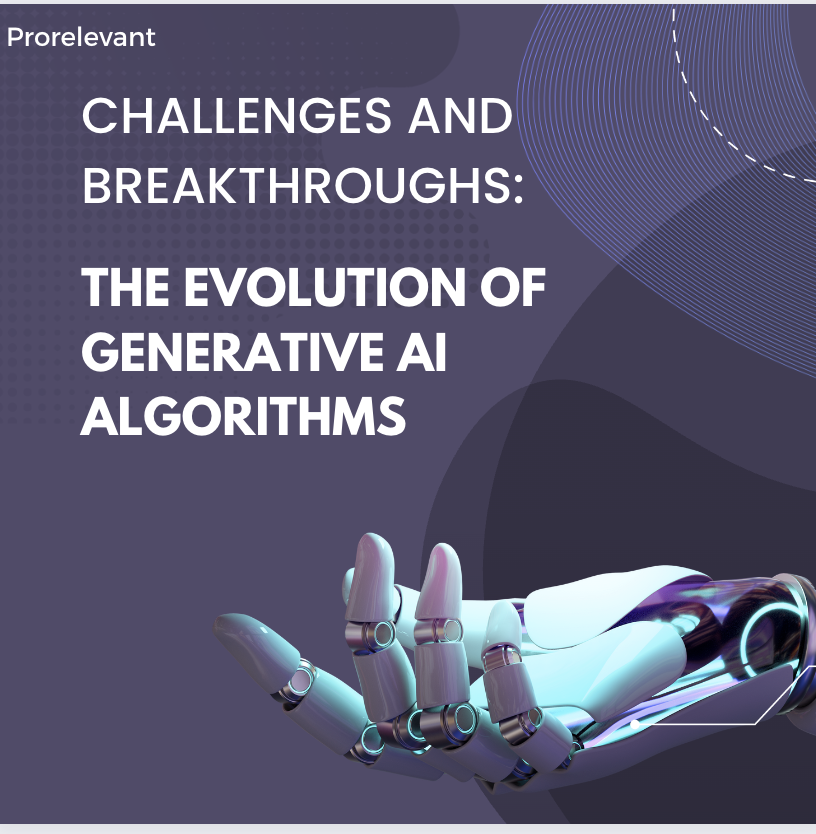
30 Sep Challenges and Breakthroughs: The Evolution of Generative AI Algorithms
Generative Artificial Intelligence (AI) has come a long way since its inception, with remarkable advancements driven by relentless research and innovation. These algorithms, which create new data samples or content, have witnessed numerous challenges researchers have tirelessly worked to overcome. This article considers the evolution of generative AI algorithms, looking at three key challenges and the corresponding breakthroughs that have propelled the field forward.
- Limited Data and Mode Collapse:
One of the initial challenges in generative AI was the reliance on limited datasets. Early models needed help to produce diverse and high-quality content. A common issue known as “mode collapse” occurred when models generated similar samples repeatedly, lacking creativity and diversity. This challenge was especially evident in Generative Adversarial Networks (GANs).
Breakthrough 1: Progressive GANs
Progressive GANs, introduced by Karras et al. in 2017, tackled this issue head-on. These networks start with a low-resolution image and progressively increase the resolution during training. This approach encourages the generation of diverse and high-quality photos. By incrementally growing the network’s complexity, Progressive GANs mitigate mode collapse and produce more realistic content. This breakthrough laid the foundation for various applications, from generating real human faces to stunning landscape imagery.
Breakthrough 2: Transformers and Text Generation
In text generation, transformers like OpenAI’s GPT-3 have revolutionized natural language generation. These models use self-attention mechanisms to capture long-range dependencies in data, enabling them to produce coherent and contextually relevant text. This innovation addressed mode collapse and improved the quality and diversity of generated textual content.
- Ethical Concerns:
As generative AI algorithms became more sophisticated, ethical concerns regarding their use and potential misuse emerged. The risk of generating fake news, deepfakes, and malicious content became increasingly evident, necessitating robust safeguards.
Breakthrough 3: Content Moderation and Detection
Researchers and tech companies developed content moderation and detection tools to mitigate the ethical concerns surrounding generative AI. These solutions employ machine learning techniques to identify and flag potentially harmful or misleading generated content. For instance, Google’s Jigsaw project utilizes AI to detect and filter out abusive language developed by chatbots, contributing to a safer online environment.
Breakthrough 4: GAN Disentanglement
Another significant breakthrough in addressing ethical concerns was the development of GANs with disentanglement capabilities. These models allow users to manipulate specific attributes in generated content while keeping others constant. For example, StyleGAN2 introduced the concept of “style mixing,” enabling users to control the hairstyle of a forged face independently of other features. This level of control empowers users to create content responsibly and ethically.
- Real-World Applicability:
While generative AI made impressive strides in research labs, transitioning these advancements to real-world applications presented challenges.
Breakthrough 5: Generative AI in Creative Industries
Generative AI has found its footing in creative industries like art and music. Artists and musicians have embraced AI tools that assist in the creative process. For instance, AI-generated artwork by artists like Mario Klingemann and AI-generated music compositions by AIVA have gained recognition and appreciation. These applications showcase the potential of generative AI in augmenting human creativity.
Breakthrough 6: Generative AI for Healthcare
Generative AI has significantly contributed to medical imaging and drug discovery in healthcare. Models like Variational Autoencoders (VAEs) have been employed to generate synthetic medical images for training and validation. Additionally, generative AI algorithms have accelerated the drug discovery process by predicting the molecular properties of potential compounds, saving time and resources.
Breakthroughs in AI Will Continue for Years to Come
Notable challenges and breakthroughs have marked the evolution of generative AI algorithms. Researchers and innovators have relentlessly pushed the boundaries of what these algorithms can achieve, from addressing mode collapse and ethical concerns to enhancing training efficiency and real-world applicability. As we progress, generative AI will continue to shape various industries and pave the way for new and exciting applications.
Want to know more?
Want to know more? Visit prorelevant.com to find out how you can customize your marketing efforts.
Check out my previous articles about Demystifing Analytic AI and The Intersection between Generative and Analytic AI.
References
Marr, Bernard. “Artificial Intelligence Explained: What Are Generative Adversarial Networks (Gans)?” Forbes, Forbes Magazine, 18 June 2019, www.forbes.com/sites/bernardmarr/2019/06/12/artificial-intelligence-explained-what-are-generative-adversarial-networks-gans/?sh=13398c807e00.
“Variational Autoencoders (VAES): Generative AI i – Synthesis AI.” Synthesis AI – The Data Generation Platform for Computer Vision, 2 June 2023, synthesis.ai/2023/02/07/generative-ai-i-variational-autoencoders/.
No Comments